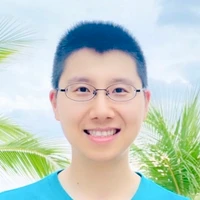
Bio Link B E R T large
michiyasunagaIntroduction
BioLinkBERT-large is a transformer-based model, pretrained on PubMed abstracts and citation link information. It enhances the traditional BERT model by incorporating document links to improve performance across several biomedical NLP benchmarks.
Architecture
LinkBERT, the architecture behind BioLinkBERT-large, is a transformer encoder that extends BERT's capabilities by integrating document links, such as hyperlinks and citation links. This approach allows the model to capture knowledge that spans multiple documents, enhancing its performance in tasks like text classification, question answering, and reading comprehension.
Training
BioLinkBERT is pretrained by incorporating linked documents into the same language model context, besides single document training. This strategy improves its ability to understand and utilize information across documents, making it effective for cross-document and knowledge-intensive tasks.
Guide: Running Locally
To use BioLinkBERT-large locally with PyTorch:
-
Install Transformers Library: Ensure you have the
transformers
library installed.pip install transformers
-
Load the Model:
from transformers import AutoTokenizer, AutoModel tokenizer = AutoTokenizer.from_pretrained('michiyasunaga/BioLinkBERT-large') model = AutoModel.from_pretrained('michiyasunaga/BioLinkBERT-large') inputs = tokenizer("Sunitinib is a tyrosine kinase inhibitor", return_tensors="pt") outputs = model(**inputs) last_hidden_states = outputs.last_hidden_state
-
Fine-tuning: For fine-tuning, utilize the LinkBERT repository or any BERT-compatible fine-tuning codebase.
Cloud GPUs
Consider using cloud services like AWS, GCP, or Azure for access to GPUs, which can significantly speed up model training and inference.
License
BioLinkBERT-large is released under the Apache 2.0 license, which allows for both commercial and non-commercial use.