Other
Text Generation
Text To Image
Text Classification
Fill Mask
Text2text Generation
Automatic Speech Recognition
Image Text To Text
Sentence Similarity
Token Classification
Feature Extraction
Translation
Image Classification
Text To Speech
Question Answering
Image To Image
Summarization
Object Detection
Image Segmentation
Image To Text
Text To Video
Audio Classification
Zero Shot Image Classification
Image Feature Extraction
Zero Shot Classification
Audio To Audio
Visual Question Answering
Reinforcement Learning
Image To Video
Text To Audio
Video Classification
Unconditional Image Generation
Time Series Forecasting
Video Text To Text
Any To Any
Audio Text To Text
Depth Estimation
Text To 3d
Image To 3d
Robotics
Mask Generation
Table Question Answering
Voice Activity Detection
Document Question Answering
Keypoint Detection
Zero Shot Object Detection
Tabular Classification
Graph Ml
Tabular Regression
More Related APIs in Sentence Similarity
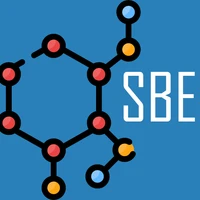
all Mini L M L6 v2
Sentence Similarity
3 months ago
81.5M
2.9K
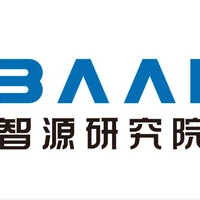
bge m3
Sentence Similarity
7 months ago
2.1M
1.7K
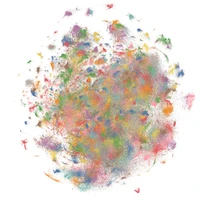
nomic embed text v1.5
Sentence Similarity
20 days ago
1.5M
522
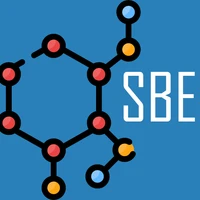
all mpnet base v2
Sentence Similarity
3 months ago
31.2M
971
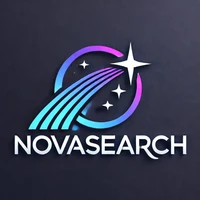
stella_en_1.5 B_v5
Sentence Similarity
a day ago
243.8K
223
B G E m3 ko
Sentence Similarity
3 months ago
8.8K
35
gte modernbert base
Sentence Similarity
13 days ago
31K
77
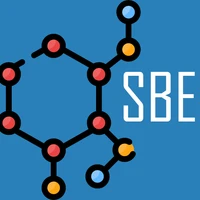
paraphrase multilingual Mini L M L12 v2
Sentence Similarity
3 months ago
9.8M
773
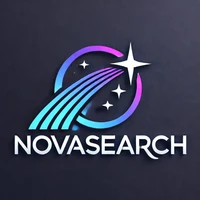
stella_en_400 M_v5
Sentence Similarity
a day ago
365.9K
180
Newsletter
Get updates on new LLM models, and other cool AI stuff.Made by Mohd Danish & Data from Hugging Face