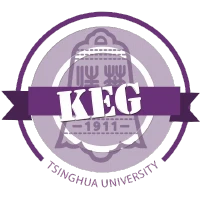
glm 4 9b chat 1m
THUDMGLM-4-9B-CHAT-1M
Introduction
GLM-4-9B-CHAT-1M is an open-source version of the GLM-4 series, developed by Tsinghua University’s Knowledge Engineering Group. This model excels in multiple domains including semantics, mathematics, reasoning, and code understanding. It offers advanced functionalities such as multi-turn dialogue, web browsing, code execution, and long-text reasoning, supporting up to 1 million tokens in context (approximately 2 million Chinese characters). It includes multilingual support for 26 languages including Japanese, Korean, and German.
Architecture
GLM-4-9B-CHAT-1M builds upon the capabilities of the GLM-4 series, featuring the ability to process extensive context lengths and perform complex language tasks. It is designed for high performance in both chat and code-related tasks and incorporates human preference alignment for enhanced interaction quality.
Training
The model has been evaluated on various datasets, showing high performance across different test areas. Long-text capabilities have been specifically tested using the LongBench-Chat and other large-context scenarios, demonstrating its robustness and effectiveness in handling extensive context lengths.
Guide: Running Locally
To run GLM-4-9B-CHAT-1M locally, follow these steps:
-
Environment Setup: Ensure that your Python environment meets the dependency requirements. Install dependencies using the provided
requirements.txt
from the GitHub repository. -
Model Execution:
-
Using Transformers:
import torch from transformers import AutoModelForCausalLM, AutoTokenizer device = "cuda" tokenizer = AutoTokenizer.from_pretrained("THUDM/glm-4-9b-chat-1m", trust_remote_code=True) query = "你好" inputs = tokenizer.apply_chat_template([{"role": "user", "content": query}], add_generation_prompt=True, tokenize=True, return_tensors="pt", return_dict=True) inputs = inputs.to(device) model = AutoModelForCausalLM.from_pretrained("THUDM/glm-4-9b-chat-1m", torch_dtype=torch.bfloat16, low_cpu_mem_usage=True, trust_remote_code=True).to(device).eval() gen_kwargs = {"max_length": 2500, "do_sample": True, "top_k": 1} with torch.no_grad(): outputs = model.generate(**inputs, **gen_kwargs) outputs = outputs[:, inputs['input_ids'].shape[1]:] print(tokenizer.decode(outputs[0], skip_special_tokens=True))
-
Using VLLM:
from transformers import AutoTokenizer from vllm import LLM, SamplingParams max_model_len, tp_size = 131072, 1 model_name = "THUDM/glm-4-9b-chat-1m" prompt = [{"role": "user", "content": "hello"}] tokenizer = AutoTokenizer.from_pretrained(model_name, trust_remote_code=True) llm = LLM(model=model_name, tensor_parallel_size=tp_size, max_model_len=max_model_len, trust_remote_code=True, enforce_eager=True) stop_token_ids = [151329, 151336, 151338] sampling_params = SamplingParams(temperature=0.95, max_tokens=1024, stop_token_ids=stop_token_ids) inputs = tokenizer.apply_chat_template(prompt, tokenize=False, add_generation_prompt=True) outputs = llm.generate(prompts=inputs, sampling_params=sampling_params) print(outputs[0].outputs[0].text)
-
-
Hardware Recommendation: For optimal performance, using a cloud GPU is recommended due to the model's computational requirements.
License
The use of GLM-4-9B-CHAT-1M’s weights is subject to the GLM-4 license. Ensure compliance with the license terms when using the model.