Other
Text Generation
Text To Image
Text Classification
Fill Mask
Text2text Generation
Automatic Speech Recognition
Image Text To Text
Sentence Similarity
Token Classification
Feature Extraction
Translation
Image Classification
Text To Speech
Question Answering
Image To Image
Summarization
Object Detection
Image Segmentation
Image To Text
Text To Video
Audio Classification
Zero Shot Image Classification
Image Feature Extraction
Zero Shot Classification
Audio To Audio
Visual Question Answering
Reinforcement Learning
Image To Video
Text To Audio
Video Classification
Unconditional Image Generation
Time Series Forecasting
Video Text To Text
Any To Any
Audio Text To Text
Depth Estimation
Text To 3d
Image To 3d
Robotics
Mask Generation
Table Question Answering
Voice Activity Detection
Document Question Answering
Keypoint Detection
Zero Shot Object Detection
Tabular Classification
Graph Ml
Tabular Regression
More Related APIs in Text Classification
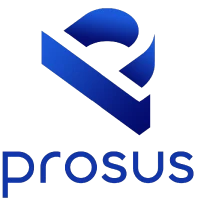
finbert
Text Classification
2 years ago
913.4K
758
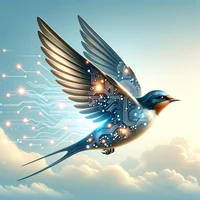
edu classifier
Text Classification
7 days ago
76
8
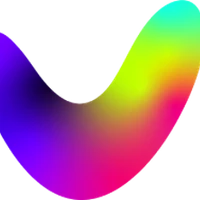
hallucination_evaluation_model
Text Classification
3 months ago
133.7K
245
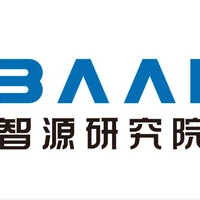
bge reranker v2 m3
Text Classification
7 months ago
889.7K
484
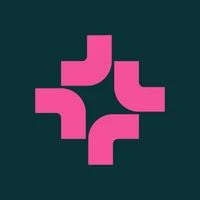
Llama 3.1 Tulu 3 8 B R M
Text Classification
7 days ago
11.3K
16
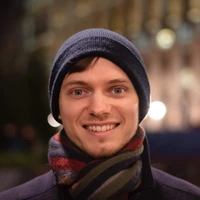
Modern B E R T large zeroshot v2.0
Text Classification
21 days ago
7.9K
38
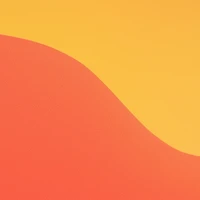
distilbert base uncased finetuned sst 2 english
Text Classification
a year ago
6.6M
681
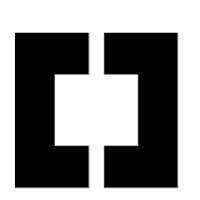
multilingual sentiment analysis
Text Classification
21 days ago
59.1K
53
Guard Reasoner 1 B
Text Classification
4 days ago
90
4
Newsletter
Get updates on new LLM models, and other cool AI stuff.Made by Mohd Danish & Data from Hugging Face