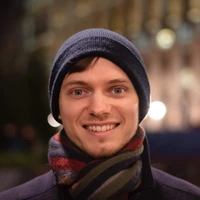
m De B E R Ta v3 base xnli multilingual nli 2mil7
MoritzLaurerIntroduction
The mDeBERTa-v3-base-xnli-multilingual-nli-2mil7 is a multilingual model designed for natural language inference (NLI) across 100 languages, suitable for zero-shot classification. It is based on the mDeBERTa-v3-base model pre-trained by Microsoft and fine-tuned on various NLI datasets.
Architecture
This model leverages the DeBERTa architecture, which stands out for its performance in multilingual contexts, especially in natural language inference tasks. It was fine-tuned using over 2.7 million hypothesis-premise pairs in 27 languages.
Training
The model was trained on datasets like multilingual-NLI-26lang-2mil7 and XNLI, incorporating hypothesis-premise pairs across 26 languages. The training process employed specific hyperparameters, such as a learning rate of 2e-05 and a batch size of 32, over three epochs.
Guide: Running Locally
- Setup Environment: Install the required packages using pip:
pip install transformers torch
- Load Model: Use the Transformers library to load the model.
from transformers import pipeline classifier = pipeline("zero-shot-classification", model="MoritzLaurer/mDeBERTa-v3-base-xnli-multilingual-nli-2mil7")
- Perform Inference: Provide a sequence and candidate labels for classification.
sequence_to_classify = "Angela Merkel ist eine Politikerin in Deutschland und Vorsitzende der CDU" candidate_labels = ["politics", "economy", "entertainment", "environment"] output = classifier(sequence_to_classify, candidate_labels, multi_label=False) print(output)
- Cloud GPUs: For enhanced performance, consider using cloud-based GPUs from providers like AWS, GCP, or Azure.
License
This model is licensed under the MIT License, allowing for flexibility in usage and distribution.