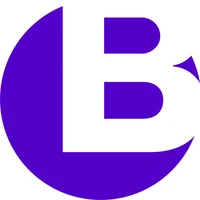
R M B G 1.4
briaaiIntroduction
RMBG v1.4, developed by BRIA AI, is an advanced background removal model designed for effective separation of foreground and background in diverse image types. It is available for non-commercial use under a Creative Commons license. For commercial applications, a separate license is required.
Architecture
The model is based on an enhanced version of the IS-Net architecture, incorporating unique training schemes and a proprietary dataset. These enhancements significantly improve the model's accuracy and performance across various image-processing tasks.
Training
RMBG v1.4 was trained using a dataset of over 12,000 high-resolution, fully licensed images with pixel-wise accuracy. The training data spans diverse categories, ensuring balanced representation across gender, ethnicity, and disabilities. The dataset includes:
- Objects only: 45.11%
- People with objects/animals: 25.24%
- People only: 17.35%
- People/objects/animals with text: 8.52%
- Text only: 2.52%
- Animals only: 1.89%
The images are split into photorealistic (87.70%) and non-photorealistic (12.30%) categories, and background types are divided into non-solid (52.05%) and solid (47.95%).
Guide: Running Locally
-
Installation:
Install the required dependencies using the following command:pip install -qr https://huggingface.co/briaai/RMBG-1.4/resolve/main/requirements.txt
-
Usage:
- Using Pipeline:
from transformers import pipeline image_path = "https://farm5.staticflickr.com/4007/4322154488_997e69e4cf_z.jpg" pipe = pipeline("image-segmentation", model="briaai/RMBG-1.4", trust_remote_code=True) pillow_mask = pipe(image_path, return_mask=True) # outputs a pillow mask pillow_image = pipe(image_path) # applies mask on input and returns a pillow image
- Loading the Model:
from transformers import AutoModelForImageSegmentation import torch import numpy as np from torchvision.transforms.functional import normalize from PIL import Image from skimage import io model = AutoModelForImageSegmentation.from_pretrained("briaai/RMBG-1.4", trust_remote_code=True) device = torch.device("cuda:0" if torch.cuda.is_available() else "cpu") model.to(device) # Prepare and preprocess image image_path = "https://farm5.staticflickr.com/4007/4322154488_997e69e4cf_z.jpg" orig_im = io.imread(image_path) orig_im_size = orig_im.shape[:2] image = preprocess_image(orig_im, model_input_size).to(device) # Inference result = model(image) # Post-process and save result result_image = postprocess_image(result[0][0], orig_im_size)
- Using Pipeline:
-
Cloud GPUs:
For optimal performance and efficiency, consider using cloud-based GPU services like AWS, Google Cloud, or Azure.
License
The RMBG v1.4 model is available under the bria-rmbg-1.4 license. It is free for non-commercial use, but commercial use requires a separate agreement with BRIA. The license details can be found here.