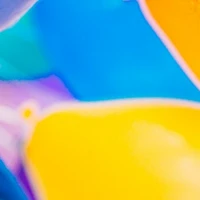
rubert tiny sentiment balanced
cointegratedIntroduction
RUBERT-TINY-SENTIMENT-BALANCED
is a fine-tuned version of the RUBERT-TINY
model, designed for sentiment analysis of short Russian texts. The task is framed as a multiclass classification problem: negative, neutral, or positive sentiment.
Architecture
The model is based on the BERT architecture and utilizes the PyTorch library. It is specifically fine-tuned for text classification tasks in the Russian language, leveraging transformers and safetensors for efficient computation.
Training
The model was trained using datasets collected by Smetanin, converted into a three-class format. The training data was balanced by upsampling and downsampling to ensure equal representation of sources and classes. The training process is documented in a publicly available Colab notebook. The model was evaluated on a balanced test set, achieving varying Macro F1 scores across different datasets, such as 0.83 on SentiRuEval2016_banks and 0.98 on mokoron.
Guide: Running Locally
-
Install Dependencies:
Ensure you have thetransformers
andsentencepiece
libraries installed:pip install transformers sentencepiece --quiet
-
Load Model and Tokenizer:
import torch from transformers import AutoTokenizer, AutoModelForSequenceClassification model_checkpoint = 'cointegrated/rubert-tiny-sentiment-balanced' tokenizer = AutoTokenizer.from_pretrained(model_checkpoint) model = AutoModelForSequenceClassification.from_pretrained(model_checkpoint) if torch.cuda.is_available(): model.cuda()
-
Inference: Use the provided
get_sentiment
function to analyze text sentiment:def get_sentiment(text, return_type='label'): with torch.no_grad(): inputs = tokenizer(text, return_tensors='pt', truncation=True, padding=True).to(model.device) proba = torch.sigmoid(model(**inputs).logits).cpu().numpy()[0] if return_type == 'label': return model.config.id2label[proba.argmax()] elif return_type == 'score': return proba.dot([-1, 0, 1]) return proba text = 'Какая гадость эта ваша заливная рыба!' print(get_sentiment(text, 'label')) # Example output: 'negative'
-
Suggested Cloud GPUs:
For optimal performance, consider using cloud GPU services such as AWS EC2, Google Cloud, or Azure.
License
The model and its associated code are shared under a license that should be verified on the official Hugging Face model page or repository. Always ensure compliance with the license terms when using and distributing the model.