Other
Text Generation
Text To Image
Text Classification
Fill Mask
Text2text Generation
Automatic Speech Recognition
Image Text To Text
Sentence Similarity
Token Classification
Feature Extraction
Translation
Image Classification
Text To Speech
Question Answering
Image To Image
Summarization
Object Detection
Image Segmentation
Image To Text
Text To Video
Audio Classification
Zero Shot Image Classification
Image Feature Extraction
Zero Shot Classification
Audio To Audio
Visual Question Answering
Reinforcement Learning
Image To Video
Text To Audio
Video Classification
Unconditional Image Generation
Time Series Forecasting
Video Text To Text
Any To Any
Audio Text To Text
Depth Estimation
Text To 3d
Image To 3d
Robotics
Mask Generation
Table Question Answering
Voice Activity Detection
Document Question Answering
Keypoint Detection
Zero Shot Object Detection
Tabular Classification
Graph Ml
Tabular Regression
More Related APIs in Text To Speech
Kokoro 82 M
Text To Speech
4 days ago
147.8K
2.8K
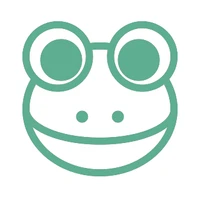
X T T S v2
Text To Speech
a year ago
2.4M
2.3K
Llasa 3 B
Text To Speech
4 days ago
6.8K
412
Llasa 8 B
Text To Speech
4 days ago
737
46
F5 T T S
Text To Speech
3 months ago
1.1M
885
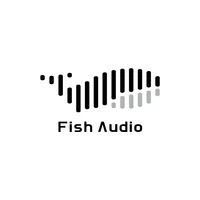
fish speech 1.5
Text To Speech
2 months ago
8.1K
421
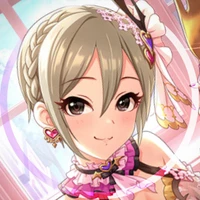
Tsukasa_ Speech
Text To Speech
3 days ago
0
37
Cosy Voice2 0.5 B
Text To Speech
a month ago
2.3K
58
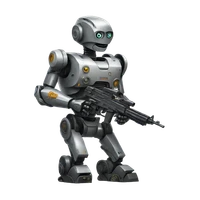
F5 T T S pt br
Text To Speech
a day ago
157
10
Newsletter
Get updates on new LLM models, and other cool AI stuff.Made by Mohd Danish & Data from Hugging Face